From Orchard Shadows to AI Insights
Every innovation begins with a vision—and the will to test it on the ground.
At the intersection of AI, agriculture, and impact stands a researcher committed to turning complex science into tangible solutions for farmers.
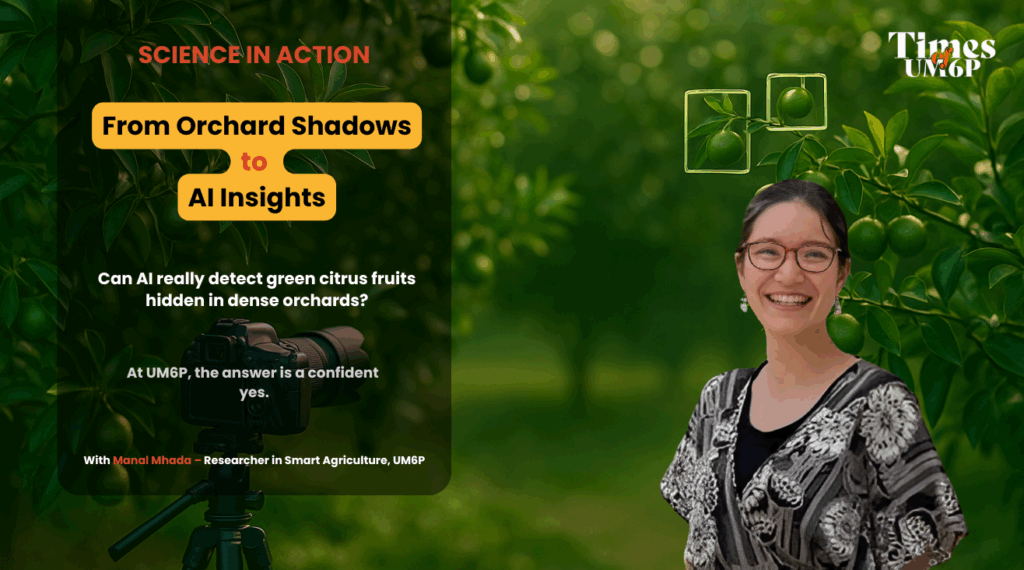
Meet Manal Mhada, the Mind Behind the Model
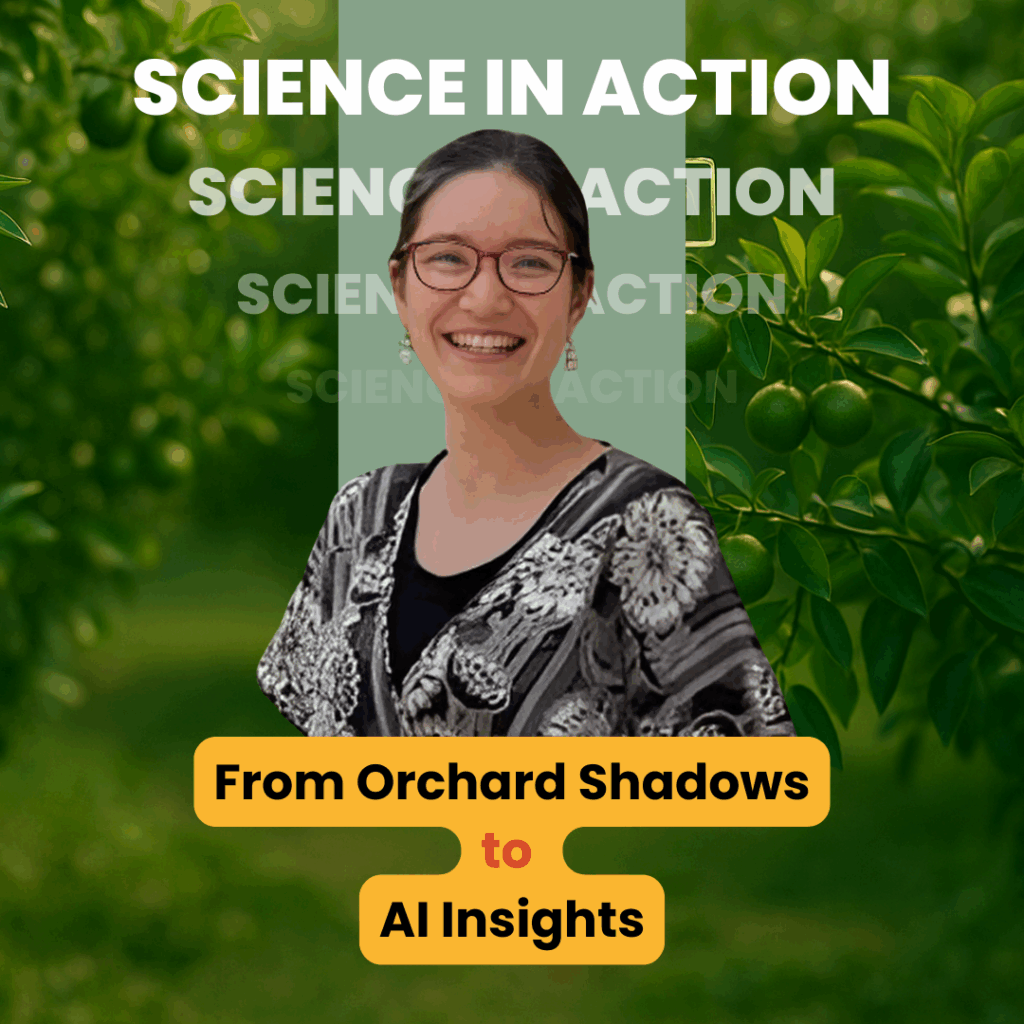
In the vibrant landscape of global agriculture, citrus fruits represent a cornerstone crop—both economically and nutritionally. Yet, detecting citrus fruits during their early, green stage poses a persistent challenge for farmers and researchers alike. These young fruits, often no larger than a walnut and camouflaged within dense foliage, escape traditional human inspection and conventional computer vision tools.
Why does this matter? Because early detection is key to estimating yields, optimizing resources like water and fertilizers, and planning timely harvests. The sooner a farmer knows how much fruit is on the trees, the more precisely they can act. But in real-world orchards, where lighting conditions vary wildly and leaves obscure the view, the task becomes a needle-in-a-haystack problem. The unripe fruits blend in so seamlessly with their leafy background that even sophisticated models struggle.
Without high-precision solutions, entire seasons may be misjudged, and decisions misinformed. At UM6P, a team of researchers led by Manal Mhada has tackled this elusive problem with one ambitious goal: teach machines to see the invisible.
How Can AI Make a Difference?
Artificial Intelligence is reshaping agriculture, empowering farmers not just with tools, but with insights. In this project, AI is not just a buzzword—it’s the brain of the operation. Deep learning models, trained on thousands of annotated images, can recognize complex visual patterns far beyond the capacity of the naked eye. When deployed on citrus trees, AI doesn’t get tired, distracted, or misled by a shadow. It detects, counts, and segments fruit systematically and at scale. What sets this project apart is its dual approach:
Close-up imagery is used to train the AI, while full-tree images—more realistic but also more complex—are used to test it. This method mimics what a farmer might actually see. By combining advanced neural networks with smart slicing techniques, the researchers overcame many challenges posed by cluttered branches and variable lighting. With this system, AI becomes a field assistant—counting fruit clusters, predicting yield, and enabling precision agriculture. And all this with just a camera and a well-trained algorithm.
Who is Behind the Breakthrough?
At the heart of this breakthrough is Manal Mhada, a researcher whose interdisciplinary vision bridges data science, agriculture, and real-world application. A member of the UM6P College of Agriculture and Environmental Sciences, Manal brings to the table not just technical rigor, but a commitment to solving pressing agricultural challenges in Morocco and beyond. Working alongside experts in plant phenotyping and machine learning, her focus is clear: leverage the latest AI technologies to serve farmers, not just papers.
Manal’s role in the project was pivotal—from coordinating field data collection to refining the deep learning pipeline and validating the model’s performance. She believes innovation must meet practicality. Our goal wasn’t to build the most sophisticated model in a lab. It was to build the most reliable solution in a real orchard. Through this work, Manal is not only elevating Moroccan agritech but also mentoring a new generation of researchers determined to make technology work for sustainability.
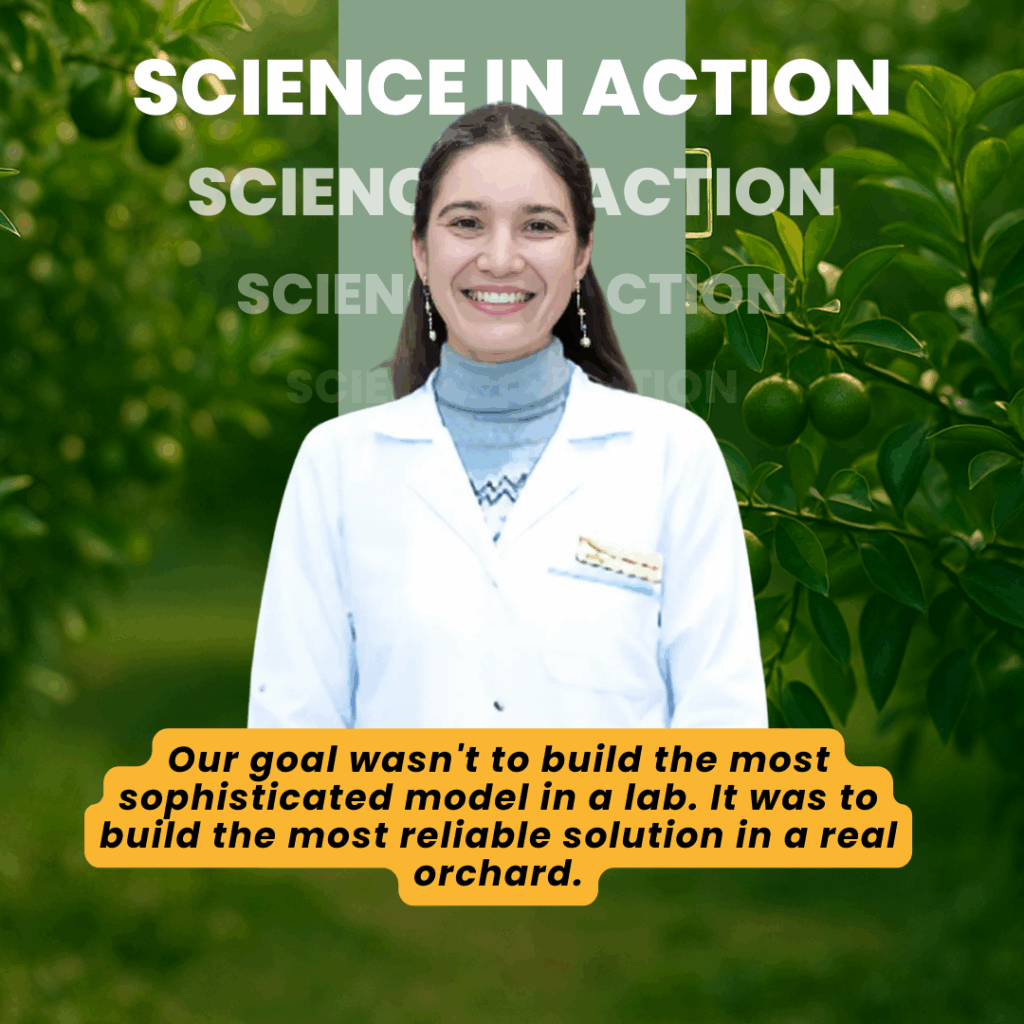
What’s the Tech Behind the Magic?
The project’s technical core is a robust framework that marries two AI powerhouses: Multiscale Vision Transformers (MViTv2) and Cascade Mask R-CNN. Think of MViTv2 as the eyes of the model, scanning the image for subtle differences in scale and color; and Cascade Mask R-CNN as the brain, refining object boundaries across stages until it reaches pixel-level accuracy. But the real innovation lies in the dual-image strategy. The system is trained on detailed close-up photos but tested on wide-angle images of full trees—where fruits are small, sparse, and often hidden.
To handle this, the researchers sliced each full-tree image into tiles, allowing the model to analyze localized sections before reassembling them into a complete fruit map. This technique not only improves detection in complex scenes but also keeps processing time efficient. Add transfer learning from massive image datasets, customized data augmentation, and optimized loss functions—and the result is a citrus-detecting AI that’s smarter, sharper, and more scalable than anything before it.
Where Does the Data Come From?
Any AI model is only as good as the data it learns from. In this case, the research team went to the field—literally. The images were captured in a commercial citrus orchard north of Marrakech, covering three distinct clementine varieties: Nules, Sidi Aissa, and Orogrande. These varieties were selected for their different flowering timelines and fruiting patterns, offering a rich spectrum of citrus phenotypes.
Two protocols guided the image collection: one with close-up shots taken just 50 to 80 cm from the fruit, and another with full-tree images shot from 3 meters away. This meticulous data collection was carried out under natural lighting, accounting for all the visual noise that complicates detection in the real world—shadows, glare, leaf occlusion, and uneven fruit sizes. Each image was manually annotated, including over 1400 segmented fruits. The result? A dataset that not only mirrors actual farming conditions but also trains the model to perform under them.
How Well Does It Work?
Numbers don’t lie—and in this case, they speak volumes. The best-performing model configuration, powered by the MViTv2_L backbone, achieved a mean Average Precision (mAP) of 84.40% for segmentation and 98.60% for bounding box detection on the test dataset. For real-world images of full trees, the slicing technique made all the difference: the model’s fruit count correlated up to R² = 0.81 with expert manual counts. That’s impressive, considering the challenges of visual occlusion and fruit clustering. Without slicing, performance dropped sharply—demonstrating the necessity of segmenting images for detailed analysis. The model also showed adaptability across different varieties and growth stages. Interestingly, the Nules variety, which flowered earliest, had the best detection rate. More slices improved accuracy but also increased the risk of double counting—so the team found the sweet spot at nine tiles per image. In sum, the model works not just in theory, but in the unpredictable, leafy chaos of Moroccan orchards.
What’s the Bigger Picture?
Beyond the pixels and performance metrics, this work has implications that ripple far beyond citrus. In a world grappling with food security, climate unpredictability, and water scarcity, precision agriculture offers a smarter way forward. The ability to count fruits early and accurately supports better yield forecasting, optimized irrigation, and data-driven crop management—all of which translate into economic and environmental gains.
For smallholder farmers, such tools could reduce losses and improve planning. For researchers, the framework opens doors to phenotyping other crops using the same dual-image logic. And for Morocco’s growing agritech ecosystem, it signals the rise of a new breed of tech-enabled solutions rooted in local realities but poised for global relevance.
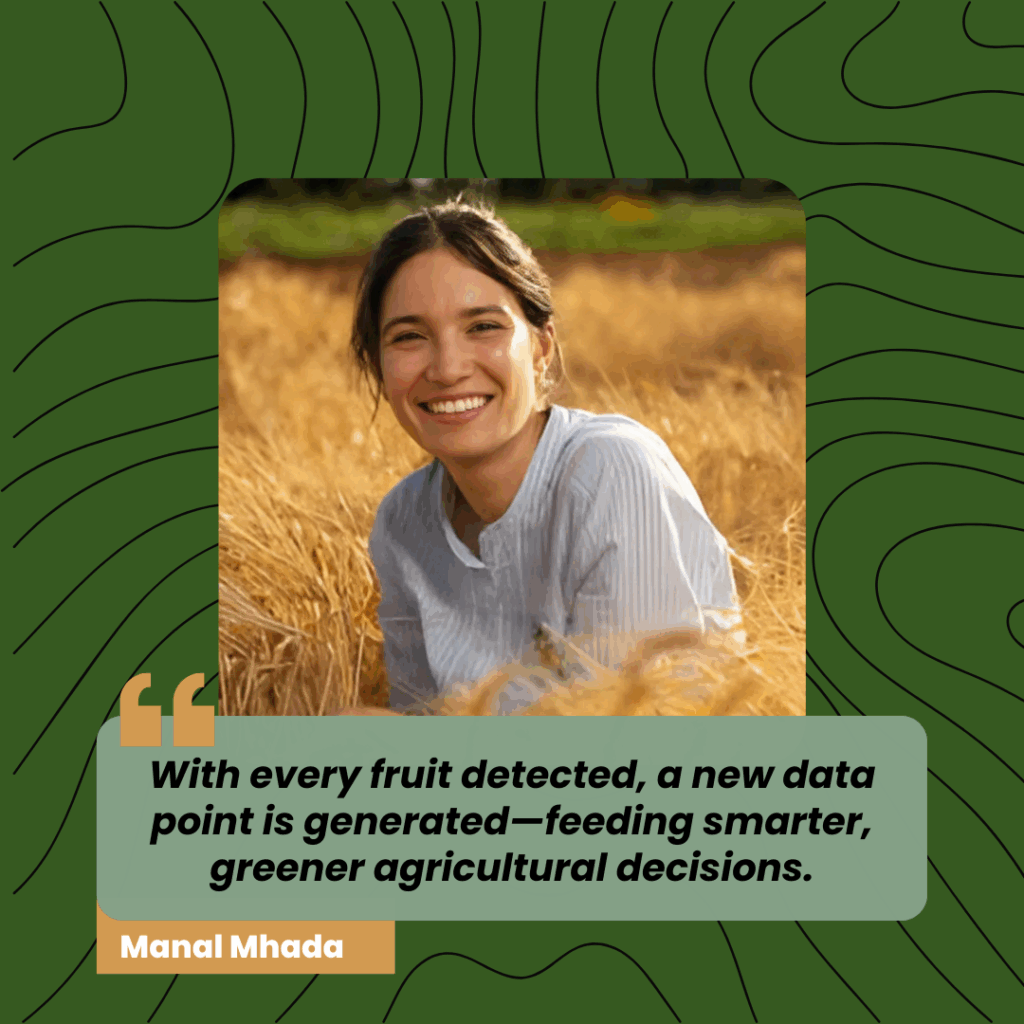
By making cutting-edge AI deployable on something as accessible as a smartphone, Manal Mhada’s team is not just solving a problem—they’re reshaping the future of farming, one fruit at a time.
What’s Next for Smart Farming at UM6P?
This project is just the beginning. With its strong base in AI, agronomy, and data science, UM6P is positioning itself as a hub for next-generation agriculture in Africa. The citrus detection framework will soon be adapted for other crops—olives, tomatoes, even cereals—each with its own set of detection challenges and benefits. The model’s lightweight design also makes it suitable for mobile deployment, bringing smart agriculture into the hands of farmers directly.
Plans are underway to integrate the framework into field apps that can guide irrigation, predict disease risk, or inform harvest timing. At the same time, the research team is exploring collaborations with international institutions to refine the model and diversify datasets. For Manal Mhada and her colleagues, the vision is clear: an agriculture that is smarter, more resilient, and driven by data—designed not only in labs but in orchards, in dialogue with farmers, and always rooted in impact.
Next Steps : Scaling Innovation Beyond Citrus
The journey doesn’t stop at oranges. The research team is now adapting the framework to detect other crops like olives, tomatoes, and even cereals—each with their own complexities. In the next 12 months, field validation trials will be launched across multiple climatic zones in Morocco, Senegal, and Ghana.
Next Steps : Scaling Innovation Beyond Citrus
🚀 Scaling Innovation Beyond Citrus
The journey doesn’t stop at oranges. The research team is now adapting the framework to detect other crops like olives, tomatoes, and even cereals—each with their own complexities. In the next 12 months, field validation trials will be launched across multiple climatic zones in Morocco, Senegal, and Ghana.
📱 Toward a Farmer-First App
A dedicated mobile app prototype is underway. Designed with local farmers in mind, it will provide real-time fruit counts, harvest alerts, and even drought risk flags. The interface will support Arabic, French, and English, and will run on basic smartphones.
🤝 Open Access & Community Science
To foster adoption and accelerate innovation, UM6P plans to publish parts of the annotated dataset and model weights on open repositories. Farmers and citizen scientists will be invited to submit photos, helping to improve the model in real-time while contributing to a shared, inclusive dataset.
A Greener Tomorrow
🌱 Agriculture with Foresight
What if farmers could know their harvest months in advance? This citrus detection system brings us closer to that vision. By reducing input waste, minimizing pesticide use, and improving labor planning, it supports sustainable intensification—growing more, using less.
💧 Data-Driven Decisions
Smart detection isn’t just about yield—it’s also about smart irrigation, pest anticipation, and climate resilience. With every fruit detected, a new data point is generated, feeding models that inform eco-friendly decisions at every step of the crop cycle.
🌍 Aligned with Global Goals
This project echoes key United Nations SDGs: from Zero Hunger (Goal 2) to Climate Action (Goal 13). It’s a local solution with global ambition—bringing AI into the hands of those most in need of innovation.
A Call for Collaboration: Shaping the Future of Precision Agriculture
🤝 We Can’t Do This Alone
UM6P is opening its doors to researchers, agritech startups, policy labs, and grassroots organizations eager to push boundaries. Whether it’s refining AI models, testing the system in new geographies, or building farmer-centric interfaces—there’s room for everyone to contribute.
💡 Pilot With Us
Have a citrus orchard? Or a tomato field? UM6P is launching an open call for partners to run real-life pilots using the system. Selected partners will receive technical support, training, and visibility in scientific and development networks.
📢 Let’s Co-Design the Future
The best agricultural tools are those shaped with farmers, not just for them. Join us in creating responsive, inclusive, and scalable agri-intelligence platforms. Let’s cultivate impact—together.
👩🔬 Meet the Scientist: Manal Mhada
Manal Mhada is a researcher at the College of Agriculture and Environmental Sciences at UM6P. With a background in agronomy and applied data science, her work bridges AI technology and real-world farming challenges. At the intersection of precision agriculture and machine vision, she is leading efforts to translate lab models into field-ready tools that empower farmers. Manal is passionate about making research more inclusive, impactful, and rooted in the needs of those who cultivate the land
👩🔬 Meet the Scientist
Want to learn more about the mind behind the model?
👉 Read Manal Mhada’s full biography
📄 Read the Full Scientific Article
AI-based framework for early detection and segmentation of green citrus fruits in orchards
👉 Click here to read the article on ScienceDirect
Leave a Reply