Illuminating a Path for Morocco, Africa, and the World
Science in Action | By Times of UM6P
The work led by El Houssaine Bouras goes beyond borders. While firmly anchored in Moroccan data and needs, the forecasting system he has developed responds to a challenge that resonates globally: how can countries manage food security in the face of climate uncertainty? In this sense, the project offers not just a tool, but a model of scientific sovereignty—where countries of the Global South produce, own, and export their own innovations. For African nations in particular, where climate vulnerability is high and forecasting infrastructure remains limited, this system represents a leap forward.
It demonstrates that with the right alliances, data, and vision, it is possible to design homegrown solutions to systemic problems. From informing cereal import policy to enabling precision agriculture and equitable resource distribution, the system’s potential applications are vast. And because it is built on open-access data and adaptable algorithms, it can be recontextualized and redeployed throughout the continent and beyond. Bouras’s work thus sends a strong message: innovation in Africa is not only possible—it is essential, exportable, and capable of reshaping how the world thinks about food resilience.
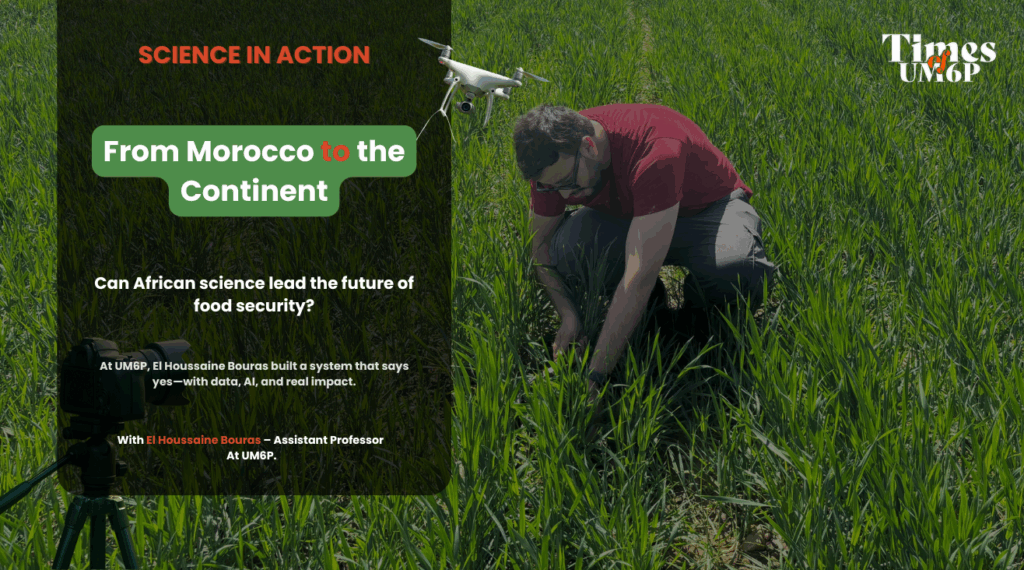
Rethinking Food Security Through AI and Climate Signals
In a world increasingly marked by climate disruption, growing cereals in Morocco has become a gamble. With over 90% of cereal production depending on rainfall, farmers, policymakers, and entire markets are at the mercy of seasonal patterns they can neither predict nor control. In this context, the question of food security transcends the boundaries of agriculture: it becomes a national priority. Recognizing this, Dr. El Houssaine Bouras and his team at UM6P
Set out to build an intelligent system that could forecast Morocco’s cereal yields several months before harvest. Their objective was ambitious but urgent: to build a tool that could empower stakeholders across the agricultural chain with early, reliable, and science-based insights. By combining satellite drought indices, local weather data, and large-scale climate indicators, they created a machine learning model capable of predicting harvest outcomes
as early as January, with remarkable accuracy. The system represents more than a technological achievement. It is a response to a systemic vulnerability—offering the ability to act before the crisis, not after. It also shows how advanced science, when rooted in local conditions and needs, can help anticipate scarcity, prevent overreactions, and guide more efficient policy and resource allocation
The Researcher Behind the Models: From Remote Sensing to Real Impact
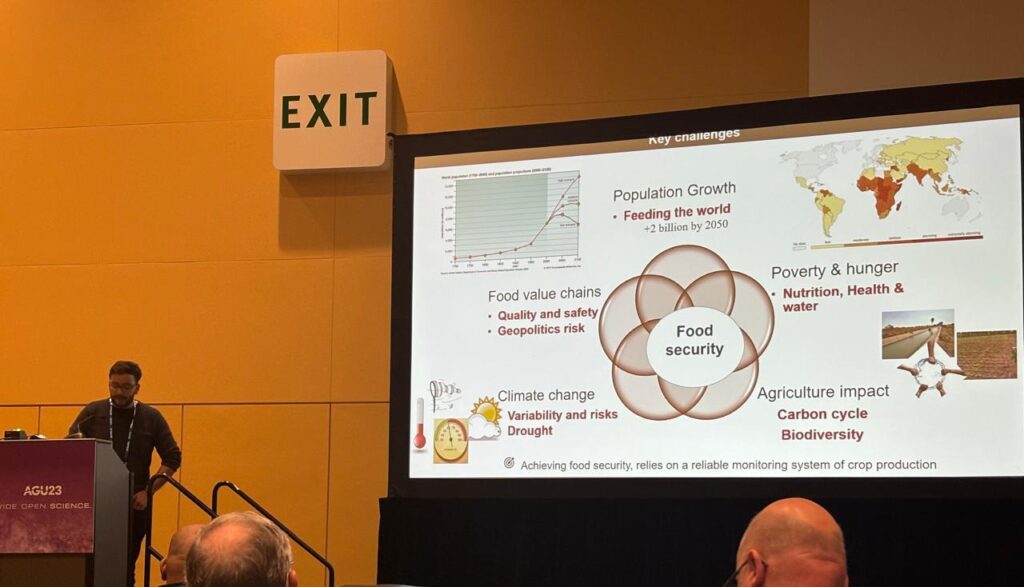
Dr. El Houssaine Bouras exemplifies the role of the modern applied researcher.
Based at the Center for Remote Sensing Applications (CRSA) within UM6P, he navigates the space between satellite science and local agronomic realities. His background in physics and environmental modeling allows him to decode how remote signals—like vegetation color on a satellite image or sea surface temperatures thousands of kilometers away—relate to cereal yield on Moroccan soil.
But his true contribution lies in application: turning complex data into tools that institutions can use. His forecasting system isn’t a closed prototype; it’s a replicable, open framework grounded in years of yield observations, rainfall and temperature data, and satellite-based drought indices. It was developed using data from Morocco’s 15 most productive cereal-growing provinces, and tested across scenarios including drought years and abnormal rainfall patterns.
Bouras champions an inclusive model of innovation—one where science doesn’t stay in academic journals but enters national dashboards, cooperative advisories, and policy briefings. By designing for impact, not just publication, he helps elevate the role of African institutions as centers of climate-smart innovation, driven by context-aware, real-world research.
How AI Reads the Fields: Inside the Predictive Engine
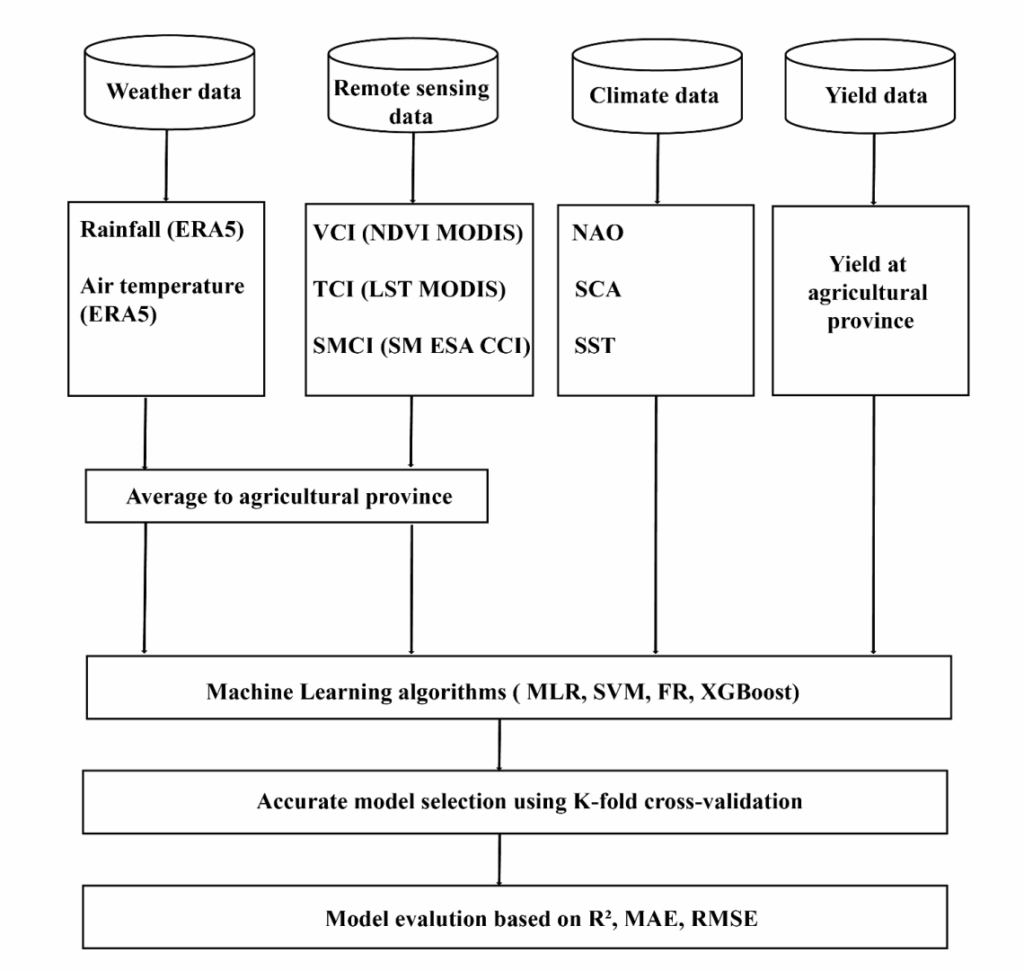
The heart of the forecasting system lies in its ability to learn—not only from the present season, but from the past two decades of Moroccan agricultural data. Bouras and his collaborators constructed a model based on three families of variables:
(1) satellite-based drought indicators such as VCI (Vegetation Condition Index), TCI (Temperature Condition Index), and SMCI (Soil Moisture Condition Index);
(2) ERA5 reanalysis weather data, covering precipitation and temperature anomalies over key growing phases;
(3) global climate oscillations including the North Atlantic Oscillation (NAO), the Scandinavian Pattern (SCA), and SST tripoles.
These variables were tested through several machine learning methods: Multiple Linear Regression, Support Vector Machines, Random Forests, and XGBoost. The latter proved most robust, yielding R² values up to 0.95 and RMSE as low as 0.20 t/ha. Each input plays a different role depending on the forecasting window: climate indices are most useful in January (four months ahead) while NDVI and SMCI dominate predictions in March, when vegetative growth reflects cumulative stress.
Unlike traditional statistical models, which assume fixed relationships, XGBoost dynamically adjusts to nonlinear interactions. It recognizes, for instance, that a warm December combined with low January rainfall might suppress yields in Settat, while the same pattern in Beni Mellal has a lesser effect due to elevation and soil properties. This nuanced capacity makes the model not only predictive but also adaptive—essential in the age of climate volatility.
Simulating Morocco: A Digital Twin for Real-World Decisions
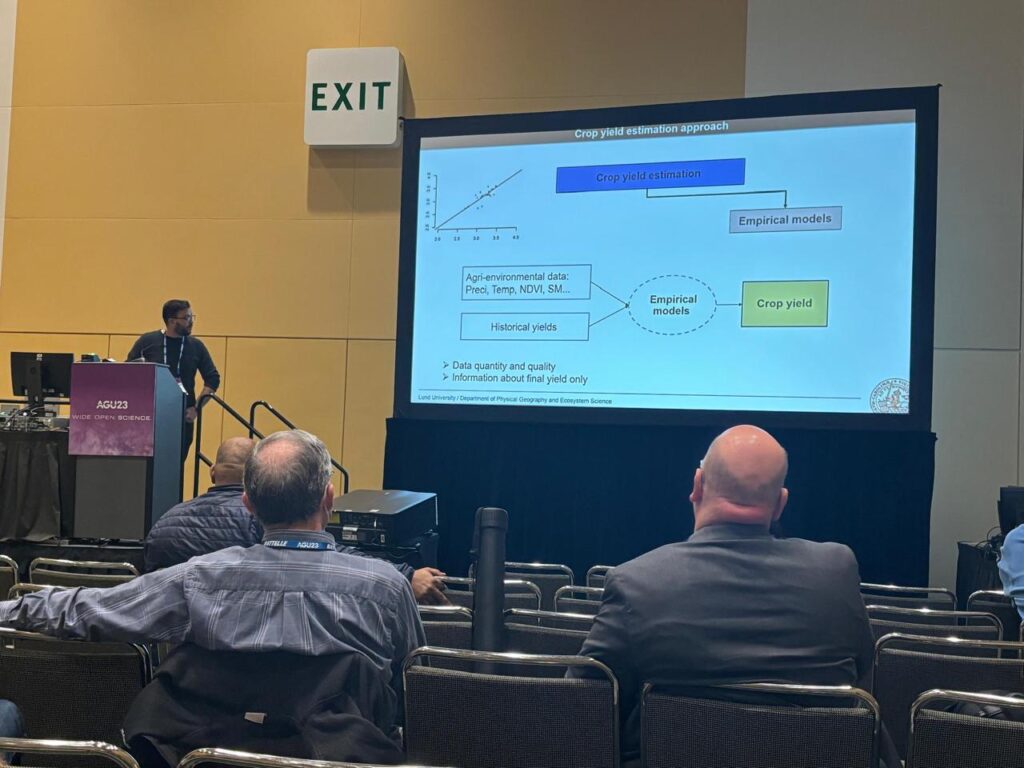
To validate the system, the team simulated 18 years of cereal yields across Morocco’s 15 key cereal-growing provinces.
Using a “leave-one-year-out” method, they trained models on historical data and tested them on untrained seasons to check real-world forecasting ability. In addition to testing general yield prediction, they classified outputs into three categories: below-normal, normal, and above-normal years.
The results showed strong performance, particularly in March where NDVI-based indices give real-time insight into vegetation stress.
But the team went further. Recognizing that Morocco is not agriculturally uniform, they introduced regional modeling: clustering provinces into zones with similar yield behavior using k-means algorithms. This approach led to a performance boost of 10–36% in RMSE depending on province. For example, coastal provinces like El Jadida responded strongly to early rainfall and showed
higher model accuracy when separated from interior plateaus.This regionalization is not just academic. It provides practical insights for policymakers: where and when to act, which inputs matter most, and how seasonal interventions can be tailored by geography. The simulation phase proved that the model could do more than analyze—it could advise.
From Data to Dignity: Why This Matters for Morocco—and Beyond
This project doesn’t just produce forecasts; it produces possibilities. In a country where agricultural productivity fluctuates wildly from year to year, the ability to anticipate yields means more than efficiency—it means dignity. Farmers no longer plant or fertilize blindly. Governments no longer wait until May to plan cereal imports. Cooperatives can distribute resources based on science, not speculation. In low-rainfall years, early forecasts enable emergency planning and prevent food stress.
In good years, they allow strategic storage and export optimization. The system also addresses broader equity gaps: many regions with sparse infrastructure can now access precision forecasting via mobile interfaces or institutional advisories.
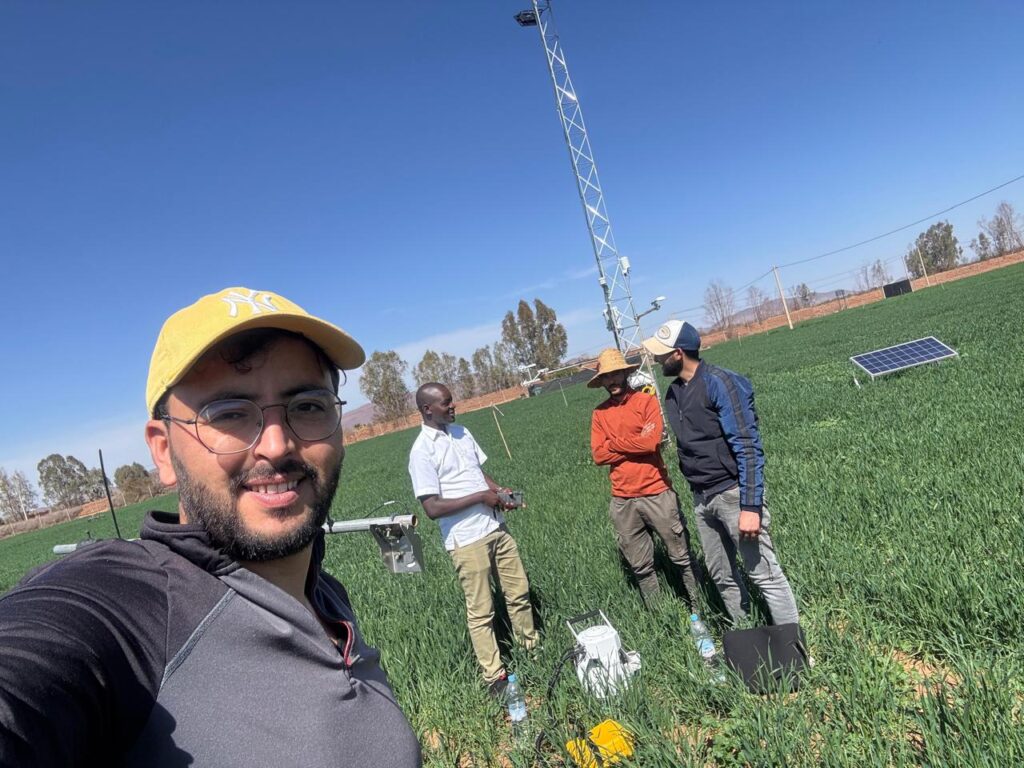
And because the tool is based on publicly accessible data and open models, it can be replicated across Africa. From Tunisia to Ethiopia, from Senegal to Sudan, any country with historical yield data and basic weather inputs can adapt the framework. This is the essence of African-led climate intelligence: not imported technology, but locally rooted, globally relevant solutions that turn vulnerability into foresight.
What Comes Next: From Research to National Infrastructure
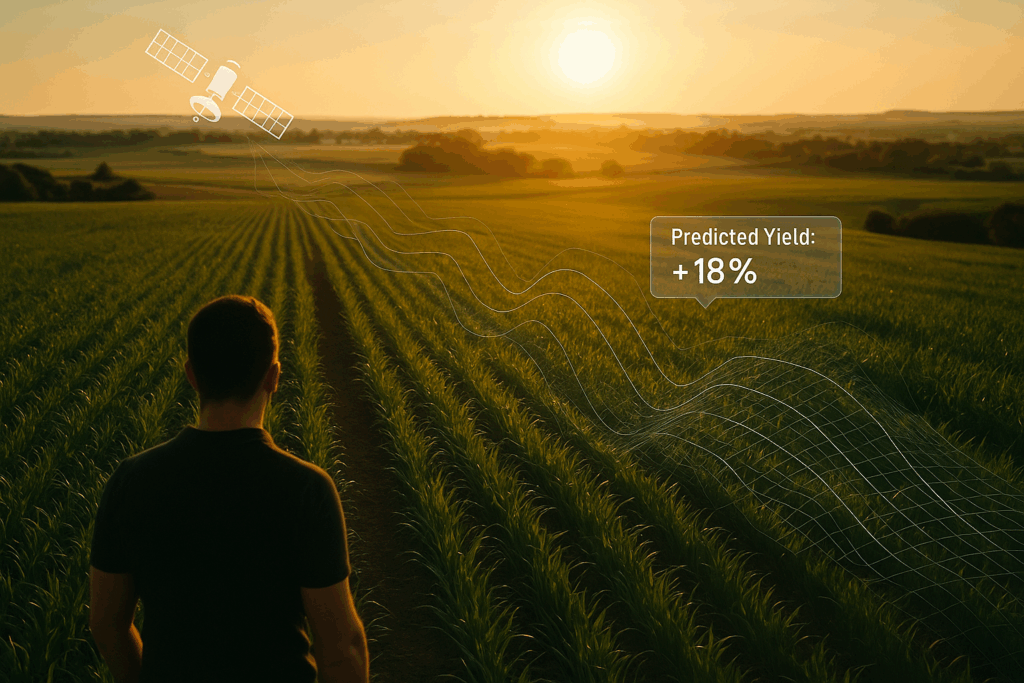
With the model validated and operational, Bouras and UM6P are now working with public institutions and international partners to embed it into Morocco’s agricultural infrastructure. Discussions are underway with national meteorological services to integrate real-time feeds. Provincial agricultural directorates are testing dashboards based on regional outputs. Development is ongoing for a mobile application that simplifies forecasts for farmers and cooperatives. Beyond Morocco, UM6P is opening access to the model’s core scripts and calibration data, facilitating replication in partner countries.
The long-term goal is to connect this forecasting engine to an ecosystem of early warning systems, market analytics, and digital advisories. In short, to make anticipation a permanent feature of African agriculture. Because in an age of climate risk, the best response is not reaction—it is readiness. And readiness begins with knowing the harvest before it happens.
A Greener Tomorrow
🌱 Agriculture with Foresight
What if Moroccan farmers could know their wheat yields months in advance? This is no longer speculative. Thanks to Bouras’s system, this vision is within reach. By reducing unnecessary inputs, guiding irrigation, and enabling adaptive planning, Morocco moves toward sustainable intensification—producing more with fewer resources, and building long-term resilience in the face of climatic stress.
💧 Data-Driven Decisions
This system goes beyond yield—it enables strategic decisions across the value chain. From real-time alerts on precipitation gaps to forecasts of regional crop failure, every satellite reading and weather anomaly feeds a model that supports more efficient, climate-aware interventions. In regions with limited infrastructure, this form of data democratization is revolutionary.
🌍 Aligned with Global Goals
This initiative supports several of the United Nations Sustainable Development Goals, including SDG 2 (Zero Hunger), SDG 13 (Climate Action), and SDG 9 (Industry, Innovation and Infrastructure). It is a Moroccan-led response to global challenges—one that offers a replicable blueprint for countries across Africa and the Global South.
A Call for Collaboration: Shaping the Future of Predictive Agriculture
📢 Let’s Co-Design the Future
Agricultural forecasting should be built with farmers, not just for them. Bouras’s team believes in participatory design—from data interpretation to interface development. Let’s build tools that are as inclusive as they are intelligent.
👨🔬 Meet the Scientist: El Houssaine Bouras
El Houssaine Bouras is an Assistant Professor of Remote Sensing and Crop Modeling at the Center for Remote Sensing Applications (CRSA) at UM6P, a collaborator with CESBIO in Toulouse, and formerly a researcher at Lund University in Sweden. His work focuses on using satellite data, AI, and climate analytics to build resilient agricultural systems. Committed to open science and regional empowerment, he works to bridge the gap between predictive models and the practical realities of smallholder farmers.
📄 Read the Full Scientific Article
Cereal Yield Forecasting with Satellite Drought-Based Indices, Weather Data and Regional Climate Indices Using Machine Learning in Morocco
🔗 Click here to read the article on MDPI
Leave a Reply